Abstract
Existing studies on fashion recommendation mainly focused on incorporating the visual signals of items to boost the user preference learning, while overlooking the semantic attributes of fashion items that also contain important cues about items' properties, e.g., material and brand, and users' preference. To bridge this gap, we aim to comprehensively explore the attribute and vision modalities of items to improve the fashion recommendation performance. However, this is non-trivial due to the heterogeneous multi-modal data, various relation types, and imbalanced attribute distribution. To address these challenges, we propose a Multi-Modal enhanced Fashion Recommendation Scheme (MM-FRec). Specifically, to cope with the multi-modal data, we introduce a relation-oriented graph as well a vision-oriented graph, and design MM-FRec with three key components: attribute-enhanced latent representation learning, visual representation learning, and multi-modal enhanced preference modeling.
In the first component, as a major novelty, to deal with the various relation types, we present a new relation-aware propagation method for adaptively aggregating the information from neighbor nodes to promote the user and item representation learning, where the deep multi-task learning strategy is introduced to alleviate the imbalanced attribute distribution issue. In the second component, we build a vision-oriented graph, i.e., a user-image bipartite graph, and based on that derive the user's and item's visual representations. In the last component, the user and item representations derived from the two modalities are fused for modeling the user's final multi-modal enhanced preference. Notably, the feasible connection paths between the users and items in the learned user-item-attribute tripartite graph can provide explanations for the prediction results. Extensive experiments on a real-world dataset demonstrate the superiority of our model over state-of-the-art methods.
Model

Framework
Dataset
To adapt IQON3000 to our task of fashion item recommendation,we treated items in an outfit composed by a user as the positive items for this user. We argue that if a user publically shares an outfit, then he/she should prefer the composing items in the outfit.To ensure the quality of the dataset, we filtered out the items that are preferred by less than 10 users. Ultimately, the final derived dataset, named as IQON10, comprises 3,568 users, 23,363 fashion items and 459,146 interactions. Table 1 lists the overall statistics of our dataset, while Table 2 shows the detailed statistics for different attribute types. As can be seen, the number of the triplets for different attribute types varies in a large range, which confirms the imbalanced attribute distribution in practice.
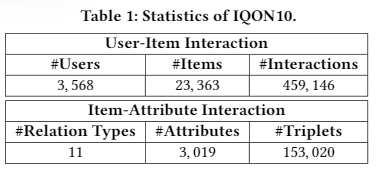
